Android SDK¶
Demo App¶
The demo APK below is built for Samsung S23 with Snapdragon 8 Gen 2 chip.
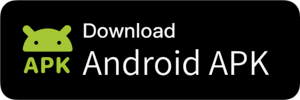
Prerequisite¶
Rust (install) is needed to cross-compile HuggingFace tokenizers to Android. Make sure rustc, cargo, and rustup are available in $PATH
.
Android Studio (install) with NDK and CMake. To install NDK and CMake, on the Android Studio welcome page, click “Projects → SDK Manager → SDK Tools”. If you have already installed NDK in your development environment, please update your NDK to avoid build android package fail(#2696). The current demo Android APK is built with NDK 27.0.11718014. Once you have installed or updated the NDK, set up the following environment variables:
ANDROID_NDK
so that$ANDROID_NDK/build/cmake/android.toolchain.cmake
is available.TVM_NDK_CC
that points to NDK’s clang compiler.
# Example on macOS
ANDROID_NDK: $HOME/Library/Android/sdk/ndk/27.0.11718014
TVM_NDK_CC: $ANDROID_NDK/toolchains/llvm/prebuilt/darwin-x86_64/bin/aarch64-linux-android24-clang
# Example on Linux
ANDROID_NDK: $HOME/Android/Sdk/ndk/27.0.11718014
TVM_NDK_CC: $ANDROID_NDK/toolchains/llvm/prebuilt/linux-x86_64/bin/aarch64-linux-android24-clang
# Example on Windows
ANDROID_NDK: %HOME%/AppData/Local/Android/Sdk/ndk/27.0.11718014
TVM_NDK_CC: %ANDROID_NDK%/toolchains/llvm/prebuilt/windows-x86_64/bin/aarch64-linux-android24-clang
JDK, such as OpenJDK >= 17, to compile Java bindings of TVM Unity runtime.
We strongly recommend setting the JAVA_HOME
to the JDK bundled with Android Studio.
e.g.
export JAVA_HOME=/Applications/Android\ Studio.app/Contents/jbr/Contents/Home
for macOS.
export JAVA_HOME=/opt/android-studio/jbr
for Linux.
Using Android Studio’s JBR bundle as recommended here https://developer.android.com/build/jdks
will reduce the chances of potential errors in JNI compilation.
Set up the following environment variable:
export JAVA_HOME=/path/to/java_home
you can then cross check and make sure$JAVA_HOME/bin/java
exists.
Please ensure that the JDK versions for Android Studio and JAVA_HOME are the same.
TVM Unity runtime is placed under 3rdparty/tvm in MLC LLM, so there is no need to install anything extra. Set up the following environment variable:
export TVM_SOURCE_DIR=/path/to/mlc-llm/3rdparty/tvm
.
Please follow Install MLC LLM Python Package to obtain a binary build of mlc_llm package. Note that this is independent from mlc-llm source code that we use for android package build in the following up section. Once you installed this package, you do not need to build mlc llm from source.
Note
❗ Whenever using Python, it is highly recommended to use conda to manage an isolated Python environment to avoid missing dependencies, incompatible versions, and package conflicts.
Check if environment variable are properly set as the last check. One way to ensure this is to place them in $HOME/.zshrc
, $HOME/.bashrc
or environment management tools.
source $HOME/.cargo/env # Rust
export ANDROID_NDK=... # Android NDK toolchain
export TVM_NDK_CC=... # Android NDK clang
export JAVA_HOME=... # Java
export TVM_SOURCE_DIR=... # TVM Unity runtime
Additional Guides for Windows Users¶
Building under Windows for Android is still experimental; please make sure you first finish the above guides, then read and follow the instructions in this section If you are using Windows, make sure you use conda to install cmake and Ninja.
conda install -c conda-forge cmake ninja git git-lfs zstd
Windows Java findings have issues with environment variables that come with space.
Make sure you get a copy of Java in a path without space. The simplest way to do that
is to copy the Android Studio’s JBR bundle to a directory without any space.
If your Android studio’s installation is at C:\Program Files\Android\Android Studio\
you can try to do the following
cp -r "C:\Program Files\Android\Android Studio\jbr" C:\any-path-without-space
set JAVA_HOME=C:\any-path-without-space
You can continue the next steps after you have set these steps correctly.
Build Android App from Source¶
This section shows how we can build the app from the source.
Step 1. Install Build Dependencies¶
First and foremost, please clone the MLC LLM GitHub repository.
After cloning, go to the android/
directory.
git clone https://github.com/mlc-ai/mlc-llm.git
cd mlc-llm
git submodule update --init --recursive
cd android
Step 2. Build Runtime and Model Libraries¶
The models to be built for the Android app are specified in MLCChat/mlc-package-config.json
:
in the model_list
, model
points to the Hugging Face repository which
model
points to the Hugging Face repository which contains the pre-converted model weights. The Android app will download model weights from the Hugging Face URL.model_id
is a unique model identifier.estimated_vram_bytes
is an estimation of the vRAM the model takes at runtime."bundle_weight": true
means the model weights of the model will be bundled into the app when building.overrides
specifies some model config parameter overrides.
We have a one-line command to build and prepare all the model libraries:
cd /path/to/MLCChat # e.g., "android/MLCChat"
export MLC_LLM_SOURCE_DIR=/path/to/mlc-llm # has to be absolute path, ../.. does not work
mlc_llm package
This command mainly executes the following two steps:
Compile models. We compile each model in
model_list
ofMLCChat/mlc-package-config.json
into a binary model library.Build runtime and tokenizer. In addition to the model itself, a lightweight runtime and tokenizer are required to actually run the LLM.
The command creates a ./dist/
directory that contains the runtime and model build output.
Please make sure all the following files exist in ./dist/
.
dist
└── lib
└── mlc4j
├── build.gradle
├── output
│ ├── arm64-v8a
│ │ └── libtvm4j_runtime_packed.so
│ └── tvm4j_core.jar
└── src
├── cpp
│ └── tvm_runtime.h
└── main
├── AndroidManifest.xml
├── assets
│ └── mlc-app-config.json
└── java
└── ...
The model execution logic in mobile GPUs is incorporated into libtvm4j_runtime_packed.so
,
while tvm4j_core.jar
is a lightweight (~60 kb) Java binding
to it. dist/lib/mlc4j
is a gradle subproject that you should include in your app
so the Android project can reference the mlc4j (MLC LLM java library).
This library packages the dependent model libraries and necessary runtime to execute the model.
include ':mlc4j'
project(':mlc4j').projectDir = file('dist/lib/mlc4j')
Note
We leverage a local JIT cache to avoid repetitive compilation of the same input.
However, sometimes it is helpful to force rebuild when we have a new compiler update
or when something goes wrong with the cached library.
You can do so by setting the environment variable MLC_JIT_POLICY=REDO
MLC_JIT_POLICY=REDO mlc_llm package
Step 3. Build Android App¶
Open folder ./android/MLCChat
as an Android Studio Project.
Connect your Android device to your machine.
In the menu bar of Android Studio, click “Build → Make Project”.
Once the build is finished, click “Run → Run ‘app’” and you will see the app launched on your phone.
Note
❗ This app cannot be run in an emulator and thus a physical phone is required, because MLC LLM needs an actual mobile GPU to meaningfully run at an accelerated speed.
Customize the App¶
We can customize the models built in the Android app by customizing MLCChat/mlc-package-config.json. We introduce each field of the JSON file here.
Each entry in "model_list"
of the JSON file has the following fields:
model
(Required) The path to the MLC-converted model to be built into the app. It is a Hugging Face URL (e.g.,
"model": "HF://mlc-ai/phi-2-q4f16_1-MLC"`
) that contains the pre-converted model weights.model_id
(Required) A unique local identifier to identify the model. It can be an arbitrary one.
estimated_vram_bytes
(Required) Estimated requirements of vRAM to run the model.
bundle_weight
(Optional) A boolean flag indicating whether to bundle model weights into the app. See Bundle Model Weights below.
overrides
(Optional) A dictionary to override the default model context window size (to limit the KV cache size) and prefill chunk size (to limit the model temporary execution memory). Example:
{ "device": "android", "model_list": [ { "model": "HF://mlc-ai/RedPajama-INCITE-Chat-3B-v1-q4f16_1-MLC", "model_id": "RedPajama-INCITE-Chat-3B-v1-q4f16_1-MLC", "estimated_vram_bytes": 1948348579, "overrides": { "context_window_size": 512, "prefill_chunk_size": 128 } } ] }
model_lib
(Optional) A string specifying the system library prefix to use for the model. Usually this is used when you want to build multiple model variants with the same architecture into the app. This field does not affect any app functionality. The
"model_lib_path_for_prepare_libs"
introduced below is also related. Example:{ "device": "android", "model_list": [ { "model": "HF://mlc-ai/RedPajama-INCITE-Chat-3B-v1-q4f16_1-MLC", "model_id": "RedPajama-INCITE-Chat-3B-v1-q4f16_1-MLC", "estimated_vram_bytes": 1948348579, "model_lib": "gpt_neox_q4f16_1" } ] }
Besides model_list
in MLCChat/mlc-package-config.json
,
you can also optionally specify a dictionary of "model_lib_path_for_prepare_libs"
,
if you want to use model libraries that are manually compiled.
The keys of this dictionary should be the model_lib
that specified in model list,
and the values of this dictionary are the paths (absolute, or relative) to the manually compiled model libraries.
The model libraries specified in "model_lib_path_for_prepare_libs"
will be built into the app when running mlc_llm package
.
Example:
{
"device": "android",
"model_list": [
{
"model": "HF://mlc-ai/RedPajama-INCITE-Chat-3B-v1-q4f16_1-MLC",
"model_id": "RedPajama-INCITE-Chat-3B-v1-q4f16_1-MLC",
"estimated_vram_bytes": 1948348579,
"model_lib": "gpt_neox_q4f16_1"
}
],
"model_lib_path_for_prepare_libs": {
"gpt_neox_q4f16_1": "../../dist/lib/RedPajama-INCITE-Chat-3B-v1-q4f16_1-android.tar"
}
}
Bundle Model Weights¶
Instructions have been provided to build an Android App with MLC LLM in previous sections,
but it requires run-time weight downloading from HuggingFace,
as configured in MLCChat/mlc-package-config.json
.
However, it could be desirable to bundle weights together into the app to avoid downloading over the network.
In this section, we provide a simple ADB-based walkthrough that hopefully helps with further development.
Enable weight bundle.
Set the field "bundle_weight": true
for any model you want to bundle weights
in MLCChat/mlc-package-config.json
, and run mlc_llm package
again.
Below is an example:
{
"device": "android",
"model_list": [
{
"model": "HF://mlc-ai/gemma-2b-it-q4f16_1-MLC",
"model_id": "gemma-2b-q4f16_1-MLC",
"estimated_vram_bytes": 3000000000,
"bundle_weight": true
}
]
}
The outcome of running mlc_llm package
should be as follows:
dist
├── bundle
│ ├── gemma-2b-q4f16_1 # The model weights that will be bundled into the app.
│ └── mlc-app-config.json
└── ...
Generating APK. Enter Android Studio, and click “Build → Generate Signed Bundle/APK” to build an APK for release. If it is the first time you generate an APK, you will need to create a key according to the official guide from Android.
This APK will be placed under android/MLCChat/app/release/app-release.apk
.
Install ADB and USB debugging. Enable “USB debugging” in the developer mode in your phone settings.
In “SDK manager - SDK Tools”, install Android SDK Platform-Tools.
Add the path to platform-tool path to the environment variable PATH
(on macOS, it is $HOME/Library/Android/sdk/platform-tools
).
Run the following commands, and if ADB is installed correctly, your phone will appear as a device:
adb devices
Install the APK and weights to your phone.
Run the commands below to install the app, and push the local weights to the app data directory on your device.
Once it finishes, you can start the MLCChat app on your device.
The models with bundle_weight
set to true will have their weights already on device.
cd /path/to/MLCChat # e.g., "android/MLCChat"
python bundle_weight.py --apk-path app/release/app-release.apk